Background
Battery-based energy storage is a great asset for the power grid, as it can respond to power imbalances very quickly. To increase profit, people try to provide multiple services with the same battery, such as fast frequency regulation, load shaving, and energy arbitrage. How to schedule these services dynamically to maximize profit is a great challenge.
Approach
Artificial intelligence comes to the rescue. We built a mathematical model that simulates a typical solar farm and its connected battery storage unit, as well as the grid and market environment it is in. Taking advantage of cutting-edge reinforcement learning techniques, we also built a PPO (Proximal Policy Optimization) agent that can interact with the model and learn how to schedule the power capacity of the battery to maximize long-term profit, taking into account the battery’s capacity fades through use cycles.
Accomplishments
Using data from SwRI’s Energy Storage Technology Center and public sources, we demonstrated that our system is safe, flexible, and quick to learn. The learning agent we chose is sample-efficient and quick to adapt to volatile market and PV (photovoltaics) generation situations. Case studies show our system beat comparative systems at maximizing long-term profit by more than 20%.
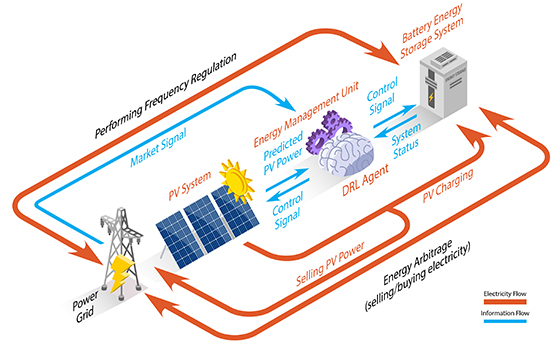
Figure 1: Overview of the machine learning model.
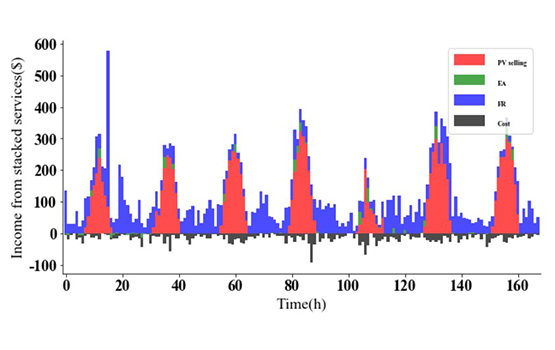
Figure 2: Revenue and cost for stacked services for a week.