Background
Over the past few years, Divisions 3 and 10 have collaborated on a variety of Connected and Automated Vehicle (CAV) technologies that improve efficiency ranging from the vehicle level [Next-Generation Energy Technologies for Connected and Automated On-Road Vehicles (NEXTCAR)] to the intersection level (Energy Efficient Mobility Systems) up to the corridor level (Integrated Corridor Management).
The overall objective of this research effort was to establish a test methodology that would integrate a dynamic and reactive traffic simulator with instrumented test vehicles to precisely measure the real-world fuel efficiency gains of CAV technologies in various traffic scenarios. This research project was conducted in two phases involving staff from Divisions 3, 8 and 10.
Approach
Phase 1 of the program focused on leveraging instrumented Class 8 trucks and demonstrating the potential fuel economy benefits of CAV technologies akin to the NEXTCAR program. The team refined the traffic simulator setup built for the NEXTCAR/Energy Efficient Mobility Systems (EEMS) program and generated additional scenarios as needed to represent a good mix or urban, suburban and highway driving. Once the virtual traffic environment was established, software-in-the-loop testing was conducted with our proprietary eco-routing, eco-driving and lane merging algorithms. Baseline and optimal speed trajectories were recorded and configured on the DEVCon system on the trucks. Track testing was conducted to quantify the fuel economy benefits on the Class 8 trucks.
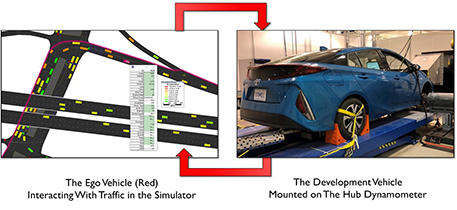
Figure 1: The Ego vehicle (Red) interacting with traffic in the simulator. The development vehicle mounted on the hub dynamometer.
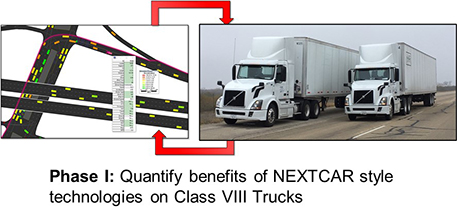
Figure 2: Phase 1: Quantify benefits of NEXTCAR style technologies on Class 8 trucks.
Phase 2 of the program focused on refining the test methodology. Specifically, the goal was to understand how to conduct closed-loop testing of vehicles on test tracks with richer scenarios enabled via such virtual traffic environments. As a first step, the team explored driver-in-the-loop testing with virtual traffic on a test track. The team then explored full automation testing with virtual traffic on a test track and investigated the impact of CAV technologies on aftertreatment systems in heavy duty trucks. Since Phase 2 of the program was more about establishing and refining a test methodology, the team leveraged the SwRI test track and SwRI-owned vehicles.
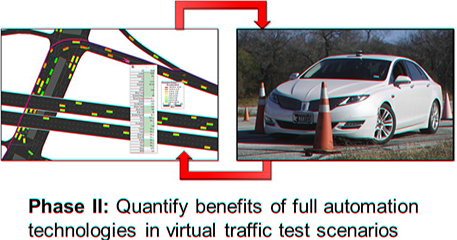
Figure 3: Phase 2: Quantify benefits of full automation technologies in virtual traffic test scenarios.
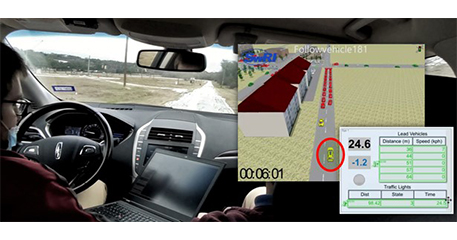
Figure 4: Integrated Digital-Twin of Automated Vehicle in Virtual Traffic Environment.
Accomplishments
Phase 1 testing with the Class 8 trucks was successful and resulted in significant improvements in fuel economy as shown in the following tables:
Table 1: Results for modified NREL Port Drayage Cycle
Test Result | |||
---|---|---|---|
Nominal | Confidence Interval | ||
Fuel Saved | 14.24% | ± | 1.81% |
Improvement | 16.61% | ± | 2.11% |
Table 2: Results for traffic simulation generated traces
Test Result | |||
---|---|---|---|
Nominal | Confidence Interval | ||
Fuel Saved | 6.83% | ± | 4.61% |
Improvement | 7.34% | ± | 4.95% |
In Phase 2, the team successfully established a virtual testing environment and integrated a digital twin of SwRI’s fully automated Lincoln MKZ to enable testing of a variety of vehicle efficiency algorithms in a mixed-reality environment.
In addition, the team evaluated real-world driving cycles on 2027+ aftertreatment systems designed for ultra-low NOx standards on production and low-NOx Class 8 engines and aftertreatment configurations. The results showed 8%-14% CO2 reduction in the eco-driving cycles and although Tailpipe NOx increased for the real-world eco-driving scenario and was above the current regulatory limit for the production system, it remained below the 2031+ HD-FTP compliance limit with the low-NOx system. The results demonstrated that eco-driving algorithms can be a technological enabler to meet current and potential future emissions targets for heavy-duty applications.