Biomechanics studies the structure and function of biological systems using mechanical principles, a discipline that dates back to the Renaissance. In the 1600s, Italian physiologist and physicist Giovanni Alfonso Borelli was the first to explain muscular movement and other body functions according to the laws of statics and dynamics.
In the 20th century, biomechanical research fueled a diverse array of advances affecting daily human life, such as the biomechanics of labor focused on increasing worker efficiency without sacrificing safety. In the world wars, biomechanics advanced prosthetics and rehabilitation medicine. Contemporary biomechanics has also advanced dentistry and orthopedics, designed safer civilian and military vehicles, and enhanced athletic prowess.

Using internal research funding, a multidisciplinary team developed human performance expertise. The initiative developed a markerless motion capture system that uses a biomechanical musculoskeletal model fused with deep learning algorithms to characterize physical motion, with results comparable to traditional marker-based systems.
A key component of biomechanics is measuring and studying human motion. Biomechanics applies the tools of mechanics and mechanical engineering to generate valuable insights into how muscles, bones and joints work together to produce motion. Applications are widespread, numerous and range from understanding and treating musculoskeletal disorders and diseases such as cerebral palsy and osteoarthritis to improving athletic performance, identifying injury risks and improving workplace ergonomics.
Conventional Motion Capture
For centuries, scientists used drawings and calculations to quantify movement. In the late 1800s, photography ushered in a new era of biomechanics analysis, but that capability was limited by manual analysis. Advancements in computer vision eventually led to 3D motion capture, starting in the 1970s. In recent decades, traditional measurement of human movement involved attaching up to 85 reflective markers to specific anatomical locations, typically at bony landmarks. These markers are tracked in 3D space as the subject performs actions such as walking. The process requires purpose-built infrared cameras, a controlled environment with consistent lighting and a complex data analysis procedure. It can take an hour to attach the markers and capture the data, followed by several days of analysis. While time-consuming and tedious, this method is considered the “gold standard” in human motion capture and analysis. Its application has significantly advanced our understanding of human movement and provided crucial insights into what happens when our musculoskeletal system fails.
However, this approach is not without limitations. The labor-intensive nature of the process along with the need for a controlled laboratory environment restricts its broader application. Additionally, placing markers on the subject can often make them self-conscious, leading to unnatural movement patterns that complicate the analysis.
Markerless Motion Capture
DETAIL
Kinematics is the branch of physics that describes movement in relation to joints and related parts.
Consider a scenario where quantifying and analyzing human movement biomechanics is as simple as observing someone in action. Southwest Research Institute developed the Engine for Automatic Biomechanical Evaluation, or ENABLE™, a markerless biomechanics software system designed to make this a reality. Unlike traditional methods, this technology enables accurate and reliable human motion quantification and automated analysis, significantly streamlining the process. The technology combines neural network algorithms, machine vision technology and biomechanical modeling to generate reliable and accurate assessments of human motion. This technology has the potential to significantly advance biomechanics, enabling widespread kinematics analysis in natural environments such as medical clinics, military training settings and athletic competitions. The applications are limited only by the imagination.
SwRI has an extremely broad and deep technical program, allowing the ability to build multidisciplinary teams to solve some of the toughest problems for our clients. This drive to collaborate led to the development of ENABLE, our markerless biomechanics technology. A chance meeting between the authors nearly eight years ago sparked brainstorming within their respective teams of biomechanical engineers, computer scientists and machine vision experts with the goal of advancing human performance research through innovative technology. Recognizing combined interests and capabilities, the team devised a plan for markerless biomechanics.
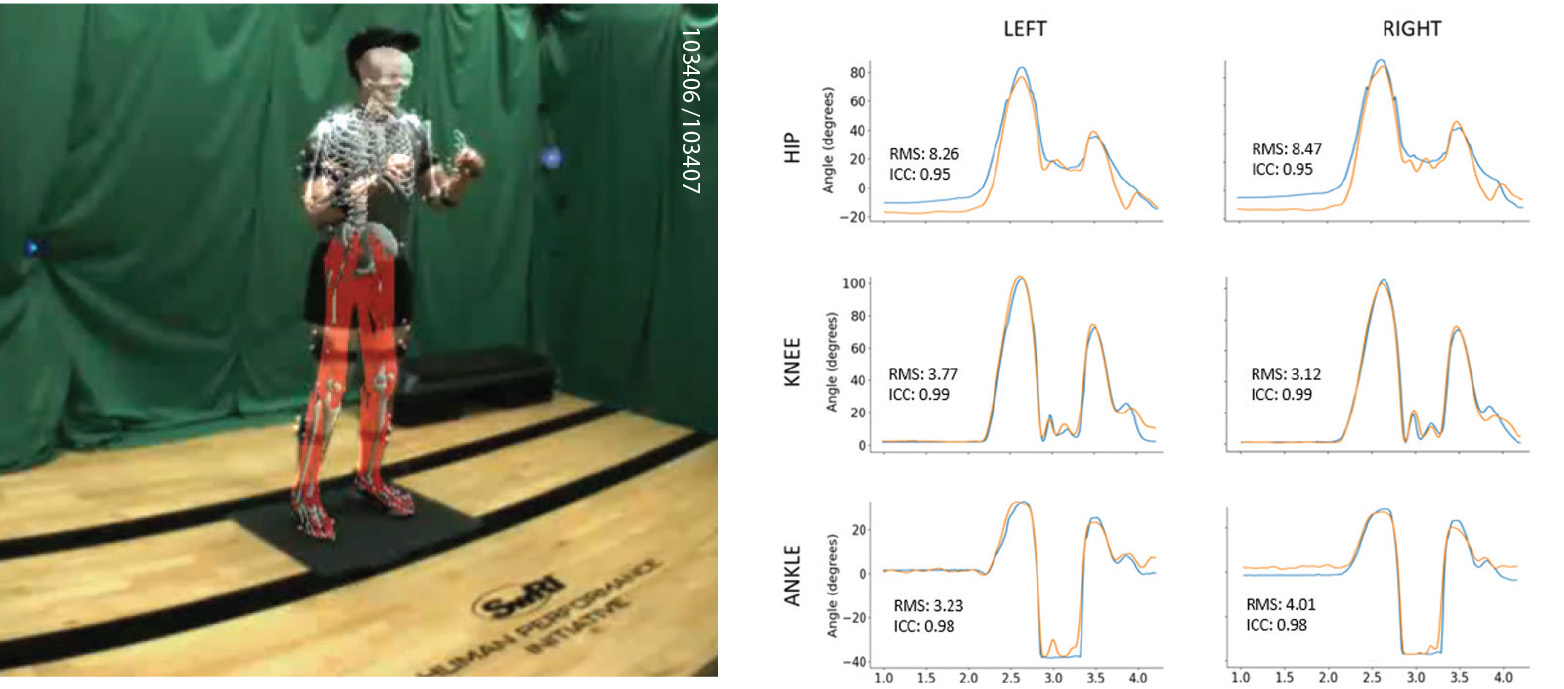
SwRI performed research and validation of its markerless biomechanics system to quantify whole body biomechanics during activities such as the counter-movement jump shown in this image.
ENABLE is a direct result of the explosive growth in artificial intelligence (AI) over the past decade, particularly in the field of machine vision and image recognition. At the heart of ENABLE is a core technology: deep neural networks. These networks, trained on thousands of labeled images, have become remarkably adept at identifying and describing what they see — for example, recognizing an Irish Setter or calico cat. ENABLE leverages this powerful technology by using AI system based on deep neural networks to identify specific anatomical points on the human body. SwRI research teaches the algorithms about the relationship between images of humans, motion and the underlying kinematics. We also developed “synthetic data,” which expanded and augmented our ability to train algorithms.
DETAIL
ENABLE, developed through SwRI’s Human Performance Initiative, estimates the underlying kinematics by analyzing video of human movement.
SwRI’s ENABLE AI is trained on a uniquely curated set of images. The team combined publicly available images commonly used for simple pose detection with video data automatically labeled through a patented method based on biomechanical modeling. The application also incorporated synthetic training data, where human avatars are precisely posed using biomechanical input data through animation. This synthetic data significantly enhances the training dataset, providing a diverse range of synthetic humans performing various activities in controlled backgrounds and environments. This capability permits rapid generation of additional training data for customized ENABLE solutions tailored to specific client applications.
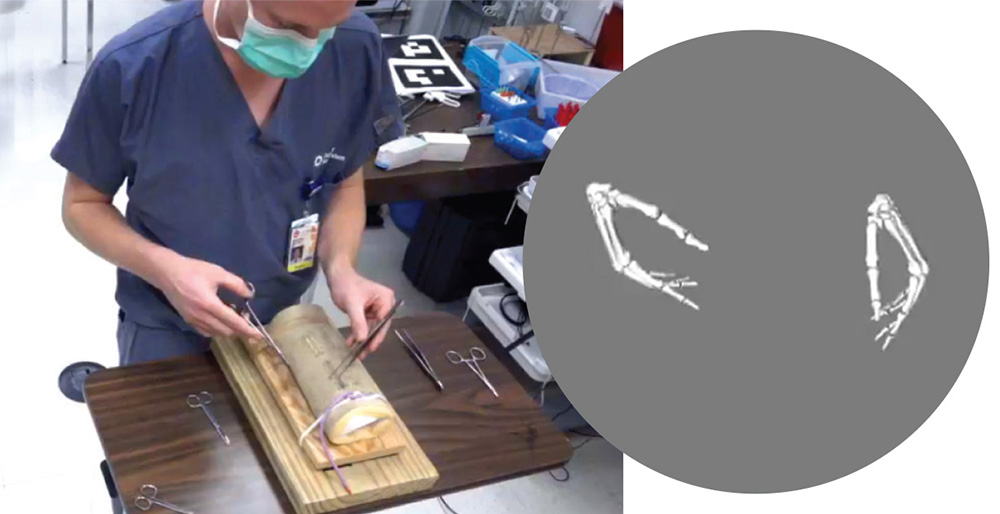
Courtesy of SwRI/Dan Nicolella/U.S. DoD
SwRI’s markerless biomechanics system was used to assess medical trainee performance on specific medical tasks such as suturing a wound.
ENABLE operates as a multicamera system, with each camera capturing the subject from a different angle. ENABLE’s underlying AI identifies virtual markers on the subject within each camera’s view in motion. Both intrinsic and extrinsic calibration data are used to reconstruct 3D trajectories of these markers as the subject moves.
The deep integration of biomechanical modeling into the ENABLE technology ensures biomechanical consistency. ENABLE automatically creates a digital twin — an accurate virtual representation — of each subject based on an OpenSim biomechanical model. This digital twin epitomizes the subject’s anatomical structure, ensuring that the output from ENABLE remains biomechanically consistent and tailored to that individual.
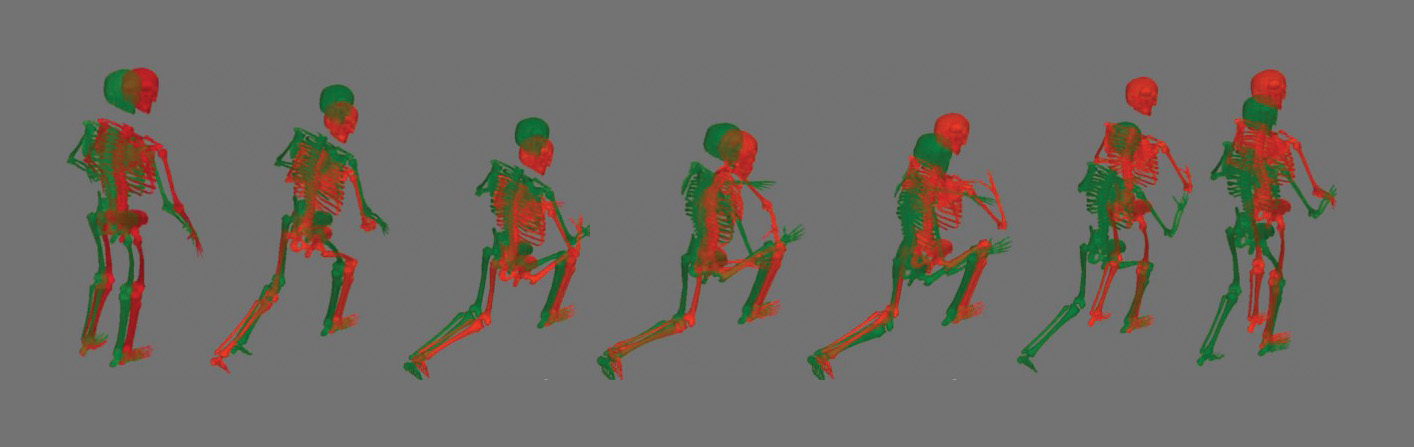
ENABLE identified musculoskeletal injury risks in military service members during specialized training. In this image, the motions of the red figures are at high risk of injury, while the green figures are at low risk.
Applications & Impacts
To validate the quantitative performance of ENABLE, SwRI collaborated with an academic research team to conduct a study comparing it to a traditional marker-based system during drop vertical jumps. The study included 135 participants, with both systems capturing 3D kinematics along with ground reaction forces. ENABLE, which was trained using a combination of real and synthetic data, demonstrated impressive performance. Root mean square errors (RMSE) for joint angles were as low as 2.52 degrees, with most results under 10 degrees — comparable to marker-based systems. The ability to characterize the torque joints experience, derived from kinematics and ground reaction forces, also showed strong agreement, with discrepancies within 0.23 Nm/kg of the marker-based system. These findings validate the accuracy of the ENABLE system and underscore the utility of synthetic training data in enhancing the performance of SwRI’s deep neural network.
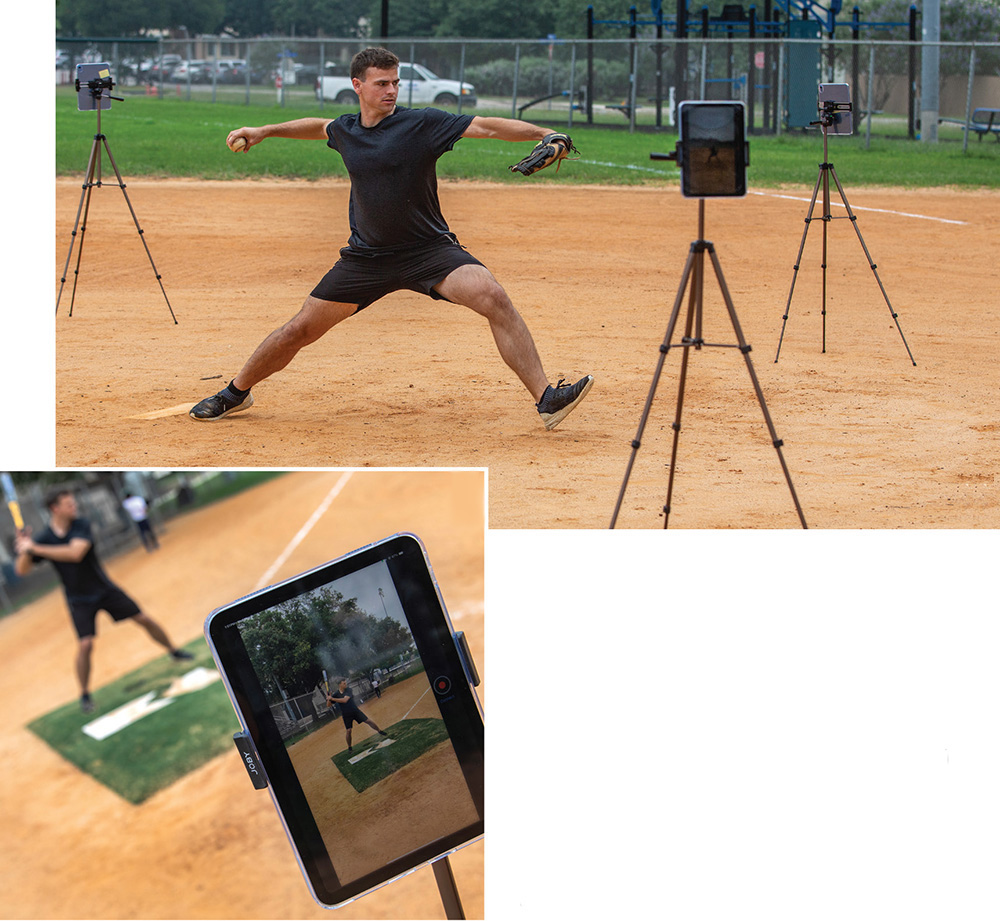
SwRI used internal funding to adapt ENABLE into a portable, user-friendly system. ENABLE is camera-agnostic, meaning it can work with video from virtually any camera system. A standalone application can wirelessly control off-the-shelf cameras, including GoPros and tablets, allowing for easy camera setup and configuration and enabling biomechanical data capture in almost any environment.
SwRI then used an early version of ENABLE to help the U.S. Department of Defense (DoD) assess medical trainees performing training exercises. Military medical training typically relies on subjective human evaluations where feedback may vary among trainers. ENABLE helped both instructors and trainees to objectively observe performance of a specific task, providing both a quantitative score, based on expert task performance, and task-specific feedback to improve performance.
DETAIL
SwRI trained ENABLE using both publicly available and custom-created datasets. To supplement this limited resource, the Institute developed its own computational toolset to generate “synthetic data.” The toolset compares and converts results from a statistical kinematic model with animation software, character models and other software to generate reliable synthetic data.
Applying SwRI’s technology to DoD medical training will allow complex assessments of 3D kinematic performance. The project assesses the detailed performance of trainees as they suture wounds and provide other combat and hospital care requiring precise hand movements or physical orientations to improve military healthcare training. Automated assessments based on SwRI-developed machine learning used actual data collected from ideal physical performance of specific medical tasks.
Moving into the athletic performance arena, SwRI has used ENABLE for a baseball pitching and basketball shooting characterization and optimization, particularly addressing performance and injury risk. Research focused on factors associated with excellent performance as well as lower risk of injury. SwRI collaborated with The University of Texas at San Antonio to capture the movements of more than a dozen pitchers, collecting video data for markerless analysis, optical marker-based motion capture for comparison, and ground reaction forces, which are the forces exerted by the ground on the body when in contact with it.
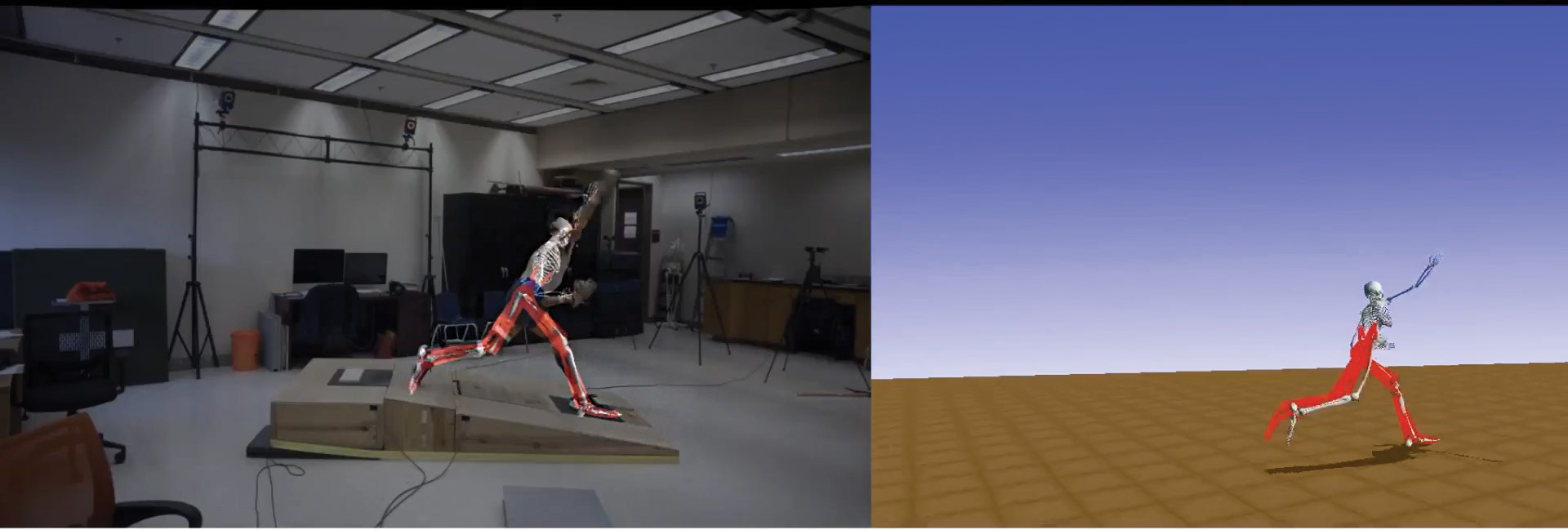
ENABLE allows biomechanical analysis of various sports and training exercises.
In addition to tracking pitcher movements, the team sought to identify potential changes in mechanics that would improve performance or reduce risk of injury. For example, researchers found that a straightened stride leg more efficiently transfers energy into the pitch. The team ran optimization scenarios to make subtle changes to pitching motion and mechanics to minimize shoulder and elbow torque, the metrics for injury, while maximizing hand velocity, the metric for performance. Then the team started looking at the biomechanics of basketball shooting and ball tracking in terms of make, miss and rim location. The team captured data for 50 subjects performing 30 shots each — 10 free throws, 10 mid-range shots and 10 three pointers.
DETAIL
OpenSim is a freely available, user-extensible software system allowing users to develop models of musculoskeletal structures and create dynamic simulations of movement.
Recently, SwRI applied ENABLE to assess musculoskeletal injury risks in military service members during specialized training. In collaboration with the U.S. Air Force Special Warfare Human Performance Squadron, SwRI used ENABLE to analyze video of more than 150 U.S. Air Force trainees performing multiple functional movements to identify trainees at risk based on their movements, aggregating the body’s entire motion. ENABLE’s predictive model correctly predicted 70% of the injuries that occurred among trainees across an eight-week training course while accurately predicting 87% of the noninjuries. The approach offers a novel way to proactively address one of the largest noncombat burdens on the U.S. military. Further refinement and wider-scale implementation of these techniques could bring about substantial injury reductions.
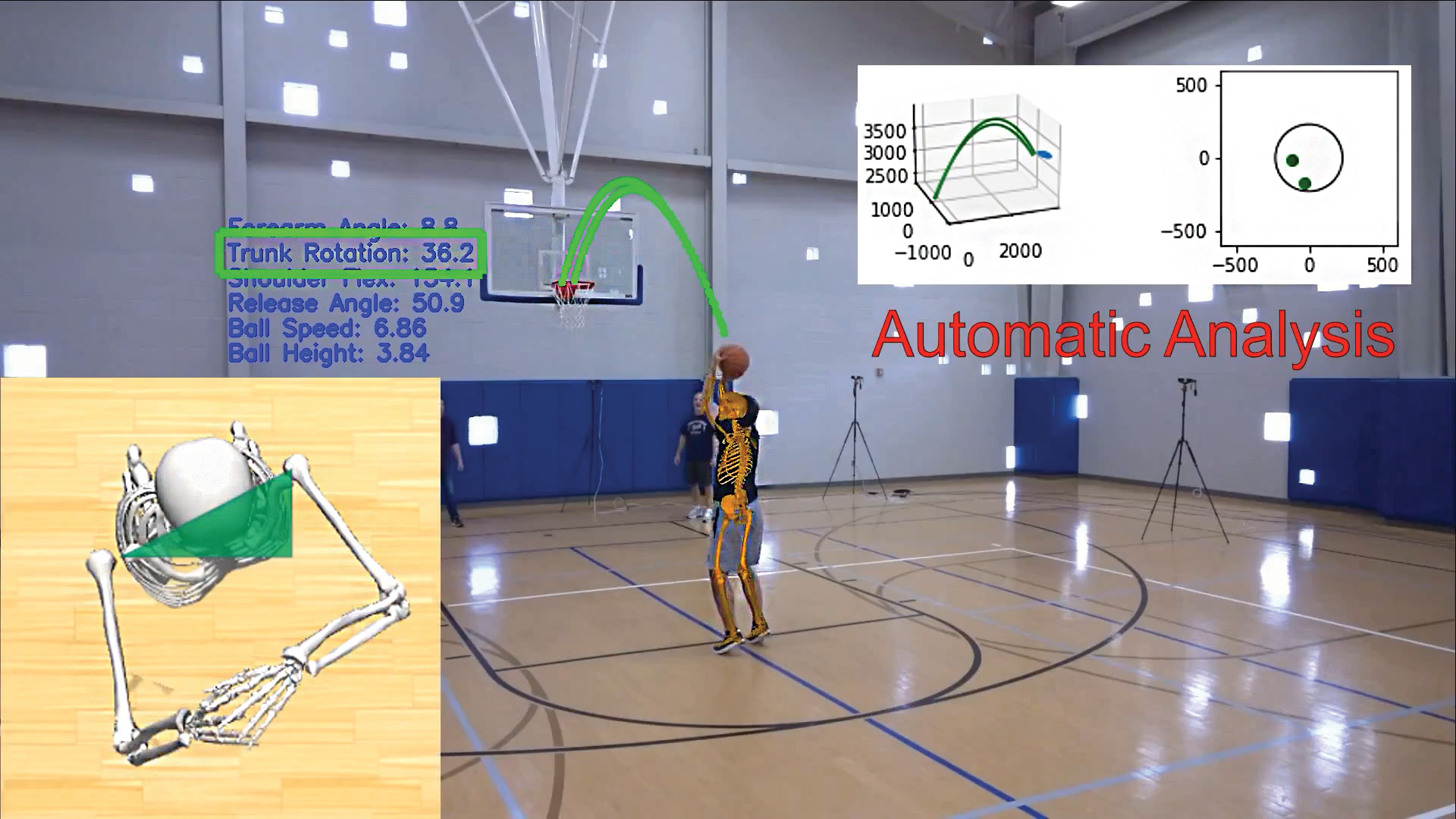
To address performance, SwRI used ENABLE to characterize and optimize basketball shooting, based on parameters such as forearm angle, trunk rotation, shoulder flex, and ball speed and height.
Conclusion
DETAIL
Noncombat musculoskeletal injuries during military training cost the U.S. military more than $3.7 billion annually.
The ENABLE markerless biomechanics system is a user-friendly and biomechanically accurate technology for capturing and quantifying human motion. ENABLE is camera-agnostic, meaning it can work with video from virtually any camera system. A standalone application can control off-the-shelf cameras, including GoPro cameras, wirelessly, allowing for easy camera setup and configuration and enabling biomechanical data capture in almost any environment. Additionally, ENABLE can serve as a “processing engine” that integrates with existing traditional motion capture systems. This capability enables existing marker-based motion capture labs to be retrofitted with video cameras and upgraded with ENABLE to achieve markerless motion capture. The technology can also support custom solutions for specific client applications. ENABLE is also available for licensing, allowing institutions and organizations to incorporate this advanced technology into their existing motion capture frameworks.
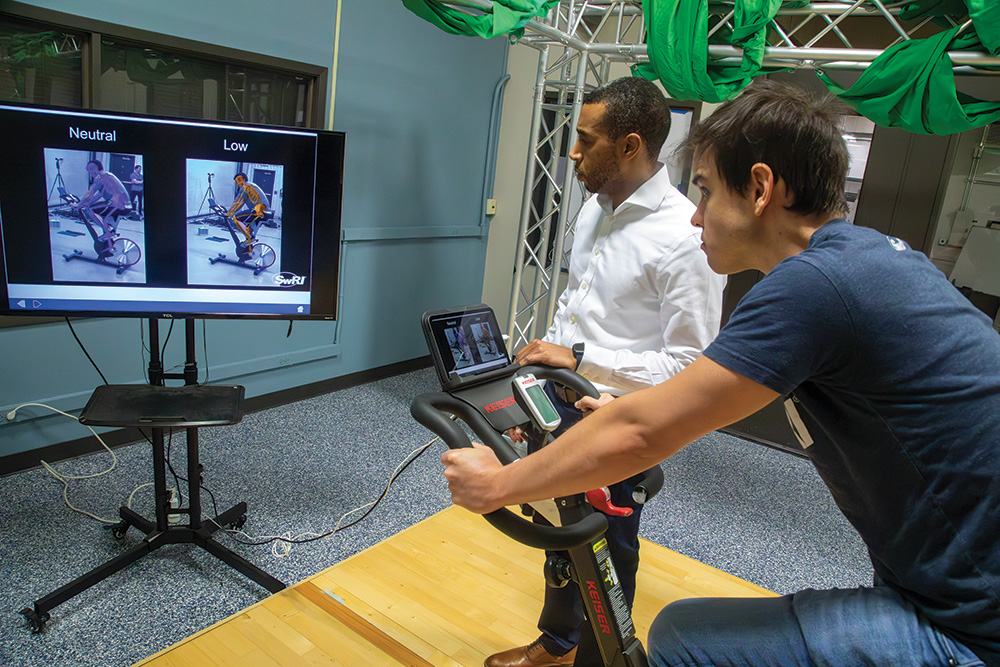
A new Human Performance Laboratory features a markerless motion capture system that allows SwRI to develop advanced biomechanical analysis tools with machine vision algorithms and data analytics.
The ENABLE markerless biomechanics system represents a significant advancement in biomechanical motion capture technology, enabling motion capture in a broad range of environments. From practical applications in healthcare, military and sports to basic and fundamental biomechanics research, ENABLE provides the capability to quickly and easily capture accurate biomechanical data in more natural environments and settings.
Questions about this story or Engine for Automatic Biomechanical Evaluation (ENABLE™)? Contact Dan Nicolella at + 1 210 522 3222 or Kase Saylor at +1 210 522 3703.
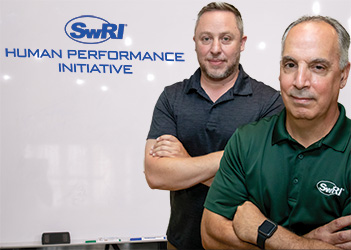
ABOUT THE AUTHORS
Dr. Dan Nicolella (right), an Institute engineer and acting manager in SwRI’s Mechanical Engineering Division, has over 30 years of experience in basic and applied research in musculoskeletal biomechanics and bioengineering. Kase Saylor is a senior program manager in SwRI’s Intelligent Systems Division, specializing in digital signal processing, embedded networks and systems engineering. Saylor and Nicolella co-lead SwRI’s Human Performance Initiative, conducting research to quantify and optimize physical performance through novel techniques and technologies.