Background
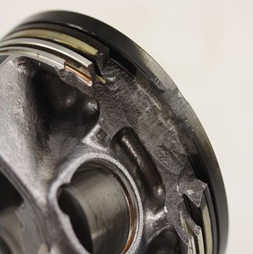
Low speed pre-ignition (LSPI), also known as stochastic pre-ignition (SPI), is an abnormal, potentially destructive form of combustion that occurs in gasoline spark-ignited engines when the air-fuel mixture is ignited prematurely. LSPI most commonly occurs in turbocharged direct-injection engines that are operating at low speeds and high loads. To meet ever increasing and challenging fuel efficiency and emission regulations, many manufacturers are developing engines that are susceptible to this phenomenon. SwRI has conducted many research initiatives in the past to identify the mechanisms that cause LSPI and has evaluated various mitigation strategies, although definitive explanations as to the cause of LSPI remain elusive.
Approach
The objective of this research was to determine if LSPI can be predicted based on macro-scale analysis across all SwRI-owned LSPI datasets. Advanced analyses, based on our extensive expertise in state-of-the-art machine learning and deep learning techniques, were used to identify whether distinct classes of LSPI exist, evaluate the basis for predictive models that can be used to detect incipient LSPI, and evaluate the feasibility of integrating these models within an engine control unit to mitigate LSPI.
Accomplishments
Data obtained as part of the Pre-ignition Prevention Program consortium was obtained, cleansed, converted, and inspected. Analyses were performed on these data to determine LSPI frequency and type among the various testing conditions. Feature importance was explored to determine which measurements have the strongest correlation with LSPI events. An automated machine learning pipeline was developed to train, evaluate, and visualize the performance of several machine learning algorithms, including decision trees, neural networks, long short-term memory (LSTM) networks, and gated recurrent unit (GRU) networks.