Background
Engine manufactures continue to be challenged with increasingly stringent emissions regulations while simultaneously being driven to improve fuel efficiency and performance. To meet these challenging requirements, new engine and after treatment technologies are continually being considered that require engineers to make complex decisions about tradeoffs between emissions, fuel efficiency, and durability. This diesel engine calibration process results in a set of software maps that is stored in the engine control unit (ECU). The number of engine actuators, sensors, and control outputs have increased over the years, resulting in a huge increase in the number of discrete test points needed to calibrate a modern engine. Industry-accepted tools such as Design of Experiments (DOE) have enabled engine calibrators to fully characterize engine operation over the entire operating range, but the huge number of data points remains an obstacle to efficiency. As such, many of SwRI’s OEM customers have voiced a desire for accelerated engine calibration activities.
The current SwRI approach to calibration is overly human-centric, requiring both a technician to run the test cell and an experienced calibration engineer to manually change variables. While passable for one- or two- variable systems common in past decades (e.g., main injection timing, rail pressure), humans have trouble optimizing the multi-variable systems that are now required to meet modern emissions regulations (e.g., main timing, pilot timing, pilot quantity, rail pressure, EGR rate, VGT position, backpressure valve). Because many of these complex interactions are non-linear, they are optimized numerically using experimental data.
Approach
For multi-variable optimization, the approach investigated here involves creating empirical neural net models of the data and then exercising the models to perform the optimization. Because the models themselves are exercised in this approach, once the data set has been taken and the models have been trained, the optimization and calibration activities can occur without taking additional data or using test cell time. An overview of this model-based calibration sequence is shown in Figure 1; the flowchart has been divided into PC-based tasks and test cell-based tasks.
Rather than designing DOEs with one piece of software, fitting models with another, and then optimizing with another, a single software was selected for investigation, namely IAV’s EasyDoE. Being a single package is hugely helpful, as the process will not require engineering labor to integrate software ad hoc; furthermore, EasyDoE natively integrates with Orion software for automated data acquisition. SwRI already owns numerous licenses of Orion. The overarching objective of this QLIR was to reduce uncertainty in the application of previously unexplored optimization techniques for engine calibration.
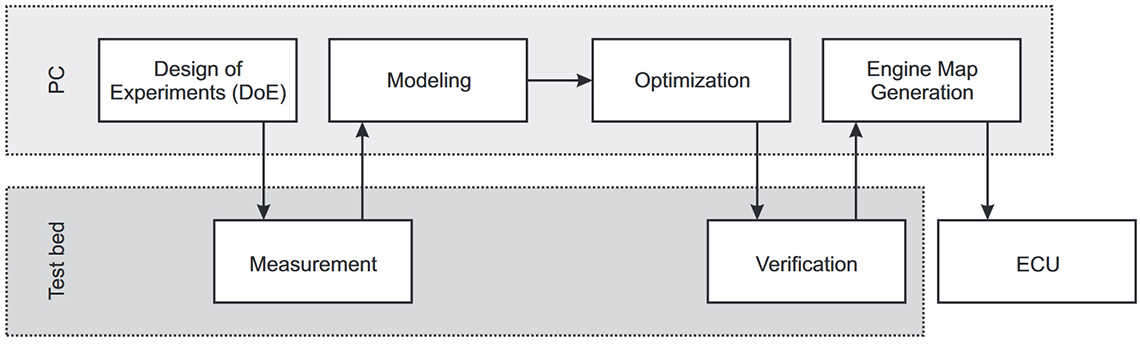
Figure 1: Flowchart of a typical model-based calibration process. SwRI is already well-versed at the test bed measurement aspect; this IR project attempted to reduce risk in the other steps, namely, DOE, Modeling, and Optimization.
Accomplishments
A new process was investigated using EasyDoE software to develop a test plan (define DOEs), create empirical models of the test cell data, and exercise the empirical models/perform optimization. EasyDoE is a software that (as hoped) can deliver the multi-variable modeling/optimization capabilities that Division 03 has been lacking. By reducing the uncertainty of this process, SwRI should now be able to more accurately and competitively bid engine development programs in the future.
- After models were fit, 9 validation points were run on-engine to compare to model predictions. Physical parameters (e.g., air flow, fuel flow, turbine inlet T) showed great correlation, but there was some error on emissions parameters. The physical parameters matching indicates that the models characterized the engine performance well and could easily meet the ± 20 percent criterion – for example, air flow and fuel flow were both within 5 percent of model predictions. However, a better controlled experiment is necessary to determine if emissions errors were due to model prediction or raw data acquisition errors.
- Compared to existing client methods of DOE design and model fitting, (estimated at 10 working days, excluding data acquisition), SwRI personnel were able to design DOEs in 2 days and fit models/optimize in 3 days using EasyDoE software. Representing an efficiency improvement of 2x, these considerable labor savings may be had if many DOE-based development programs are executed per year. It is expected that even further efficiency improvements are possible with the EasyDoE ONLINE capability. The ONLINE capability automates range finding during DOE design, and it also fits models as the data is being acquired. The latter will significantly reduce acquisition time, as only the number of points needed for good model fit are acquired. This capability was not available for investigation with the EasyDoE evaluation copy used here, so further investigation may be necessary.
- EasyDoE + ONLINE capability was quoted at $40,500. With a PL2 loaded salary, it would take 200 PL2 hours (5 weeks) to break even on the EasyDoE license. It is feasible that this threshold could be reached in the first 2 or 3 DOE-based development projects. Over an entire fiscal year, spread across a section of PL1 and PL2 development engineers, the labor time savings should easily pay for EasyDoE + ONLINE. Furthermore, the license can be leveraged by other SwRI sections when the performance and emissions calibration team is not using it, including diesel research (CHEDE), spark-ignited research (HEDGE), and large engine development.