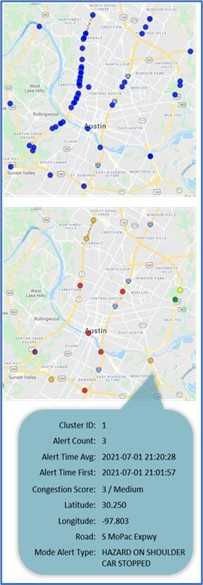
Figure 1: Before & after; clustered, weighted data provides actionable insights.
Background
Software developed by the Intelligent Systems Division helps traffic management centers (TMCs) respond to roadway incidents for swift and safe return to normal operations. Third-party and crowdsourced traffic data has potential to improve this process but generates overwhelming amounts of redundant and unreliable information to review.
Approach
Scalable serverless cloud-based technologies were used to automate collection and analysis of real-time and historical data from multiple sources. Multi-variate clustering techniques were applied to reduce crowdsourced traffic incident alerts while persisting clusters over the lifetime of an incident. Next actionable weights were calculated from third-party traffic speed data to determine whether congestion impacting travelers might require TMC operator action.
Accomplishments
The best performing algorithm reduced crowdsourced alerts by 90%, matched 89% of historical TMC managed traffic events, and reported alerts on average 19 minutes before the associated TMC managed events were detected. Clustering crowd sourced roadway alerts and fusing it with speed data to add actionable weights to clustered information has been proven as a viable candidate for integration into the toolset used by operators at a typical TMC.
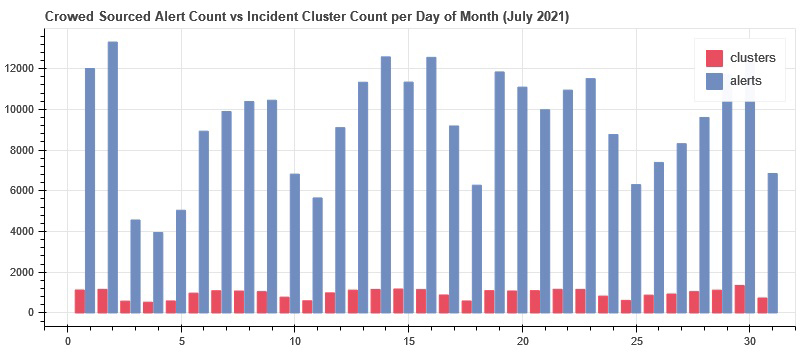
Figure 2: Average daily data was reduced by 90% (July 2021).
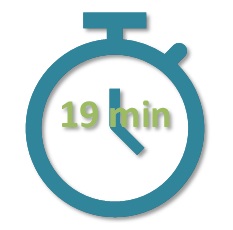
Figure 3: Average detection time was reduced by 19 minutes.