Background
Finding leaks on an oil and gas pipeline is an important task for both safety and environmental concerns. Currently, the fastest approach for inspecting pipeline right-of-ways (ROW) for leaks is to use aerial inspection services, which are conducted by a single pilot who must divide their attention among multiple tasks simultaneously. Performance during the ROW inspections vary from observer to observer, and observers rapidly become fatigued and distracted while performing airborne inspection tasks. Pipeline operators and inspection companies are looking to offload these observation and recognition tasks by using dedicated processing boards, computer vision, and machine learning to reduce operator fatigue while increasing the reliability, efficiency, and accuracy of airborne pipeline inspections.
Approach
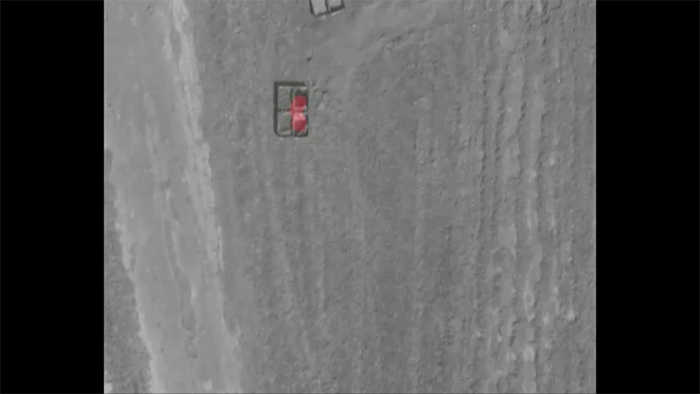
Figure 1: Video from UAS with machine learning algorithms highlighting in red the detection of crude oil on 18" x 48" sod.
The existing Smart Leak Detection (SLED) system consists of visible and longwave infrared (LWIR) cameras feeding a semantic segmentation algorithm to detect crude oil on ground and water surfaces. The SLED algorithm has been focused on detection of dark crude oils from a stationary application between 30 and 50 feet from the source. The goal of this targeted research and development project was to adapt and improve Southwest Research Institute’s (SwRI) SLED crude oil detection algorithm to work on aerial imagery and prove the feasibility of deploying machine learning-based crude oil detection systems on a fixed wing platform. These inspections are typically conducted between 500 and 1,000 feet above ground level at speeds between 70 and 140 mph.
Accomplishments
Over the course of this project, we collected 56 different configurations of crude oil on both sod and gravel for a total of 244,000 frames of data. Using two out of the nine unmanned arial systems (UAS) collection tests as holdout data, we achieved an accuracy rate of 70%. An example is shown in Figure 1.