Background
Musculoskeletal injuries (MSKIs) are a significant concern within the U.S. military, accounting for up to 70% of injuries among recruits and active soldiers. A substantial portion of these injuries occur during basic combat training (BCT), a ten-week program aimed at building foundational fitness. During BCT, approximately 25% of men and 50% of women experience some form of MSKI. Notably, a significant subset of MSKIs in BCT consists of bone stress fractures, with the tibia being one of the most common injury sites. Consequently, it is crucial to assess tissue-level mechanics at various stages of BCT as they are an important indicator of MSKI risk. To accomplish this, there is a need for a framework that can rapidly create a personalized finite element (FE) model specific to each individual, capable of predicting tissue mechanics and, in turn, injury risk. The primary objective of this Targeted Internal Research (IR) project was to develop an integrated methodology for generating a personalized, multiscale, musculoskeletal digital twin from video footage of a subject, allowing the prediction of tissue-level mechanics while the subject performs a specific movement of interest.
Approach
The objective of this IR was achieved in three steps. First, we used SwRI’s markerless motion capture system ENABLE TM to collect data from individuals doing a one-legged hop. The output of this step is a scaled OpenSim rigid body dynamics model along with the subject’s kinematics. This model is then used to perform a musculoskeletal analysis in the second step, solving for joint reaction and muscle forces using a static optimization method. Finally, a FE analysis is performed to predict the strain in the tibia. The boundary conditions and loading profiles from the second step serve as an input to this last step. Each step in this pipeline and transfer of data between steps is processed automatically with no user manipulation. The process is outlined in Figure 1. This framework is validated through experimental data from literature.
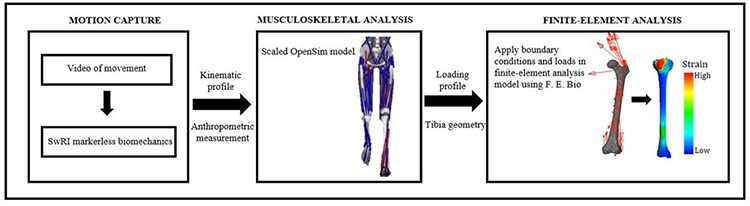
Figure 1: Procedure to go from video to strain in three steps - motion capture, musculoskeletal analysis, finite element analysis.
Accomplishments
The automated framework to go from video data to tibial strain was successfully developed and demonstrated using one-legged hopping as a validation case. The developed approach transfers the boundary conditions and loading profile from the OpenSim model to the FE model in less than a minute as opposed to a few hours if done manually. The model correctly predicted the ranking of the responses, i.e., tension highest, followed by shear and compression, and the mean always fell within one standard deviation of the experimental data (Figure 2).
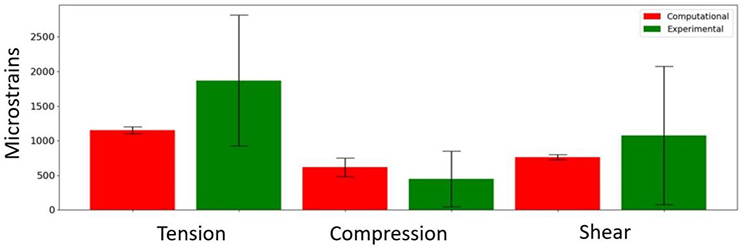
Figure 2: Comparison of peak strains, i.e. tension, compression and shear of subjects performing a one-legged hop.