Mass spectrometry (MS) generates high-quality data to identify known and unknown compounds in various samples. Mass spec data analysis provides qualitative and quantitative approaches for liquid chromatography (LC/MS), gas chromatography (GC/MS), and other separation techniques. Artificial intelligence-powered data analysis reveals insights into chemical compounds and molecular structures while offering high-quality automation to reduce time and effort to screen and analyze data.
Southwest Research Institute (SwRI) is at the forefront of methods development in mass spec data analysis, developing and applying next-generation deep learning algorithms. Our problem solvers collaborate across disciplines to develop mass spec data solutions for clients in the biomedical, pharmaceutical, environmental testing, and materials science sectors.
Artificial Intelligence Mass Spectrometry (AIMS)
SwRI’s artificial intelligence mass spectrometry (AIMS) team includes data scientists and analytical chemists with a mission to expand humankind’s understanding of the hidden molecular world. We provide simple and scalable molecular intelligence for non-targeted and targeted analysis. Our expertise in mass spec data analysis draws upon decades of excellence in analytical chemistry and computer science to develop cheminformatics machine learning solutions. With expertise from a broad spectrum of industries, we can improve chemical identification and gain insights in materials science, biology, and medicine. Our interest in emerging fields includes:
- Exposomics – Exposomics studies an individual's lifetime exposures to chemical compounds and how that affects who develops diseases and who does not. Exposures include environmental and occupational sources.
- Proteomics & Metabolics – The study of disease and proteins (proteomics) informs understanding of DNA (genomics) and its role in health-related studies of small molecules (metabolomics) and lipids (lipidomics).
Chemical Identification Lab
We characterize chemical compounds and molecular data in our chemical identification laboratory, specializing in analytical chemistry software, standardized testing, and data science, including:
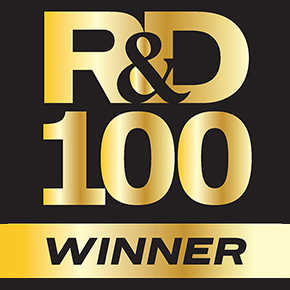
R&D 100 Award for Floodlight™ Non-Targeted Analysis System
Cheminformatics tool that analyzes mass spectrometry data recognized by R&D World as one of the 100 most significant innovations of 2021.
Learn MoreCheminformatics Machine Learning Tools
SwRI’s cheminformatics machine learning tools help clients gain insights using deep learning algorithms for mass spec data analysis.
- Floodlight Software – This cheminformatics tool features machine learning algorithms for non-targeted and targeted analysis of chemical compounds.
- Analytical Chemistry Software – Contact us to develop analytical chemistry software and cheminformatics or visit Complex Data Analysis to learn about big data solutions.
- Machine Learning Technologies