BACKGROUND
The United States Air Force (USAF) has a large fleet of aging metallic aircraft. Replacement parts for damaged structural components are required to sustain these aircraft. The military routinely has problems obtaining acceptable replacement parts for numerous reasons, including long lead time,; the original manufacturer is out of business, or fabrication processes are no longer available. This sustainment problem may be mitigated by additive manufacturing (AM) processes that can quickly produce one-off components.
However, predicting the material properties, strength, and durability of components resulting from AM processes is a complex problem. Layer-by-layer deposition of material during an AM process produces potentially severe residual stresses that distort components, introduce non-homogeneous microstructures, and alter material properties from the wrought condition. Potentially, these (and other) quantities may be tailored in the AM process based on performance requirements using integrated computational materials engineering (ICME). However, before tailoring these quantities towards a performance goal, we must first predict them accurately, and this requires a credible AM process simulation framework.
APPROACH
This project builds a computational model of the selective laser melting process and uses thermal history predictions to drive computational predictions of residual stress and average grain size. Thermal profiles for the AM process may be computed by (relatively) straightforward thermal simulations. Consider a finite element analysis with a structured grid of elements such that one grid of elements has been aligned with the build direction. In this case, elements represent powder particles that are “silent”, i.e., this element does not contribute to the thermal and/or mechanical “stiffness” of the underlying conservation equations. As a layer of powder is deposited, the elements activate and then contribute to the thermal response — primarily by conducting heat from node to node. The heat generated from the power source conducts throughout the material during the AM process. The power source may be described as either a Gaussian surface flux for mesoscale modeling or by uniform heating with an island-hopping strategy for structural-scale modeling, in which islands are contiguous element faces within a rectangular boundary.
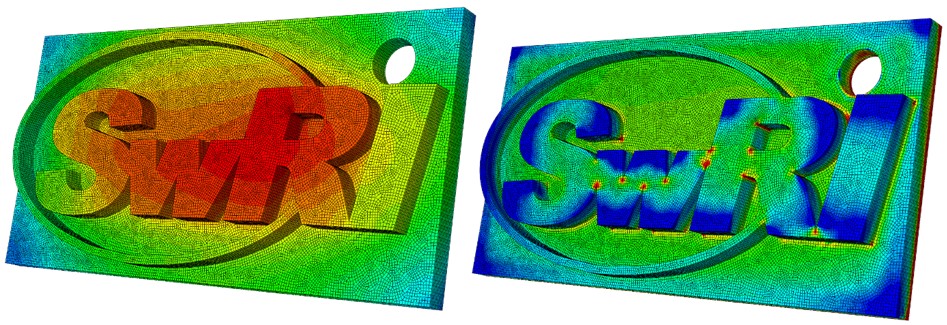
Figure 1: Temperatures and residual stresses predicted using the additive manufacturing framework developed during this project.
For residual stress calculations, consider the same finite element grid with the same mesh that was previously used in the thermal analysis. Here, the previously computed thermal histories drive material expansion that promotes residual stress development. Once again, we adopt the “active/passive” element distinction where only a layer of elements is activated at any given time, and the most recently activated layer represents powder being spread over the build platform. Passive elements do not transmit loads, whereas active elements have a load carrying capacity that reflect their thermo-mechanical response. Elements that exceed the melt temperature are relieved of their stresses. Residual stresses reflect plastic deformation that remain in the component after cooling to ambient temperature.
Microstructure evolution in an AM process is stochastic and potentially computationally intensive. However, the problem may be simplified by focusing on certain key characteristics (e.g., average grain size and shape) that are important for gross material properties (e.g., yield stress). Various researchers have had success predicting average grain size trends using energy-based, phenomenological models. These models capture the thermal effect to the grain growth by a few model parameters. They represent tractable approaches for morphology prediction.
ACCOMPLISHMENTS
Accomplishments during the first quarter of this project include capabilities to activate elements during thermal analysis; capabilities to define power source parameters and scan path; capabilities to change phase (e.g., from powder to liquid to solid); and demonstration of these capabilities on various geometries. Accomplishments during the second quarter of this project include capabilities to activate elements within a scan island; capabilities to predict microstructure (e.g., grain size); capabilities to support structural scale residual stress prediction; and demonstration of capabilities on various geometries.