Background
Reliable part reconstruction has been a challenge for the broader aerospace industry; technical issues and ineffective solutions have generated significant pull for reliable image/point cloud acquisition, and the ability to drive processes, via robust meshes, as well as execute quality assurance steps. Industry has indicated, should robust and high-quality meshes be generated, that enable reliable processing of highly reflective parts, that there is suitable business case to move forward with a production‐viable/prototype solution, of this capability, for a wide array of components, of this nature, that may be produced.
Approach
Our approach was to combine Truncated Signed Distance Function (TSDF) based reconstruction techniques, with robotic manipulators, to allow for high quality and reliable reconstruction. The project sought to implement an approach that bypasses the need for spatially dense scans, and feature‐full surfaces, by leveraging the kinematics of the robot to seed the tracking process. Furthermore, we implemented this technique with high resolution industrial 3D sensors, to produce models enabling reconstruction of very fine features that are on the order of the global accuracy of the robot.
Accomplishments
The development of mesh completeness was key to the idea that the whole part or at least the region of interest, to be processed, completely reconstructed to enable effective process planning. This was done by referencing output meshes to the known part.
Via a qualitative assessment, where Legacy methods exhibit 50-60 percent mesh completeness, and with the developed technique we realized greater than 98 percent with TSDF and greater than 99% with TSD and the Next Best View tools. However, it was clear that mesh completeness alone would not be a suitable metric to determine performance. This was in part due to the sensitivity of the selected selection.
Figures 1-4 indicate the improvement was realized with the process development sensor that was benchmarked against the prior sensor. In this case, there was sensitivity to the sensor selected, along with mesh completeness. The original sensor, that the use case and proposal was structured around, was the Ensenso N35 stereo camera, with integrated grid projection (blue light for original development and infrared for the reconstruction sensor comparison). The development sensor was the Asus XtionPro, which is an RGB sensor with an integrated depth camera that projects an IR pattern.
The improvements were noticeable, as we leveraged the TSDF and NBV processes, the sensors both leveraged stereo camera principles to develop their depth data, but in the case of the two Ensenso cameras evaluated, there was a difference in the pattern projection light wavelength and how the pattern was manipulated. This highlights the opportunity to perform more rigorous sensor selection and comparison prior to application development; however, the overall improvements to the consistency output mesh were significant over all the sensors tested.
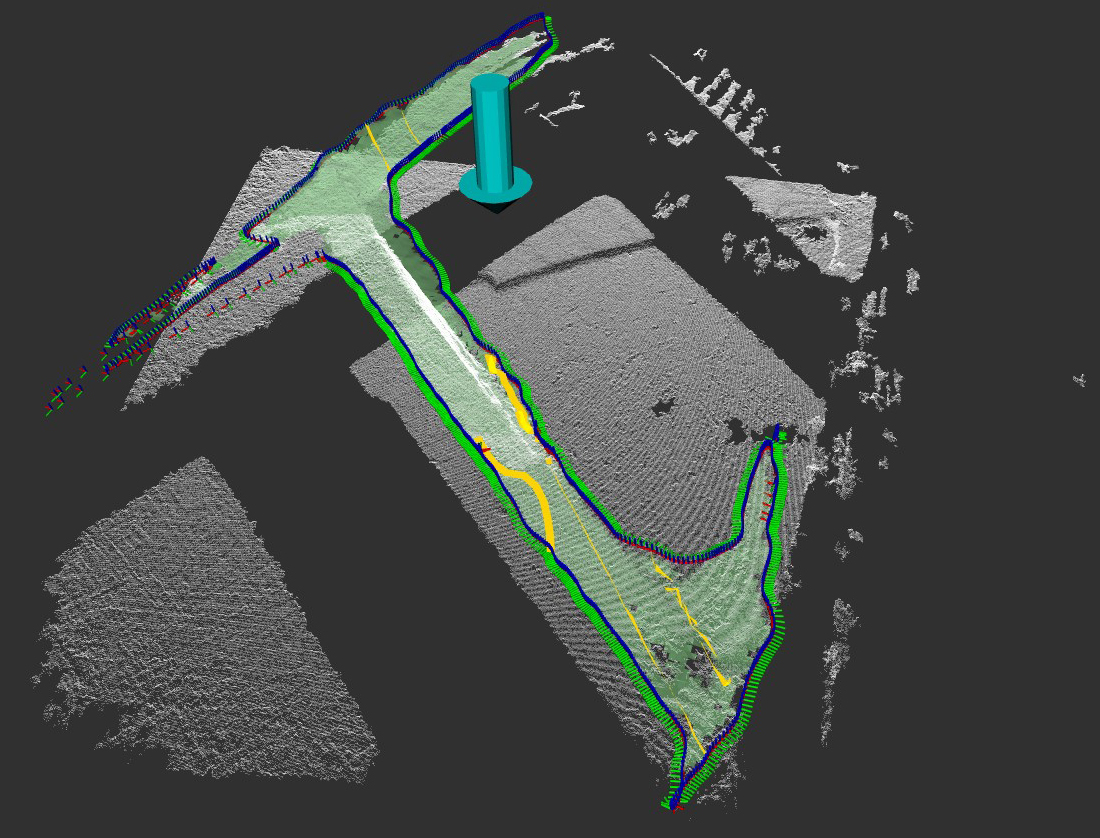
Figure 1: Original Incomplete Reconstruction with Ensenso N35 Blue Light Projection Multi-View
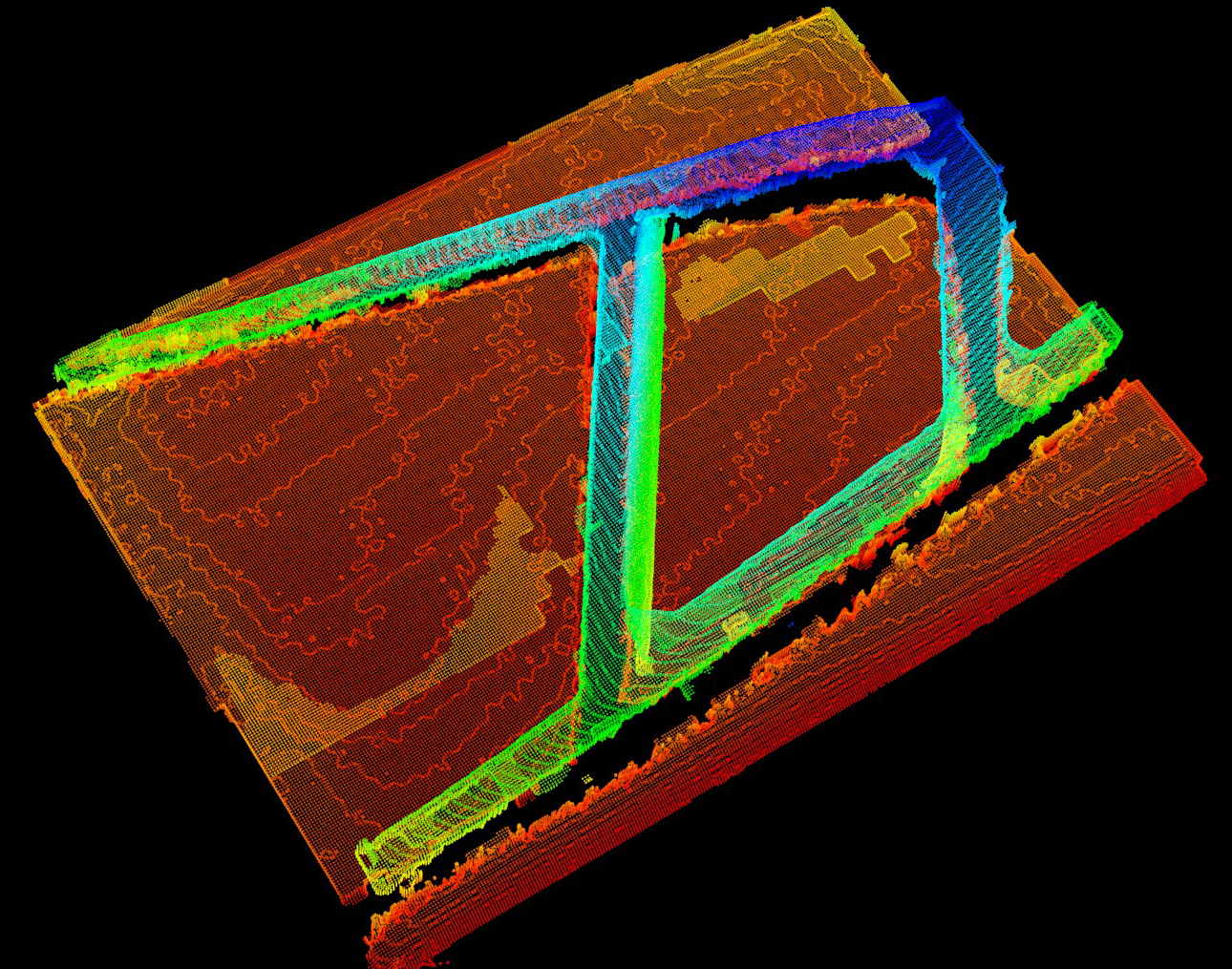
Figure 2: Developed TSDF Approach on Window Frame with Contrasting Color Mapping
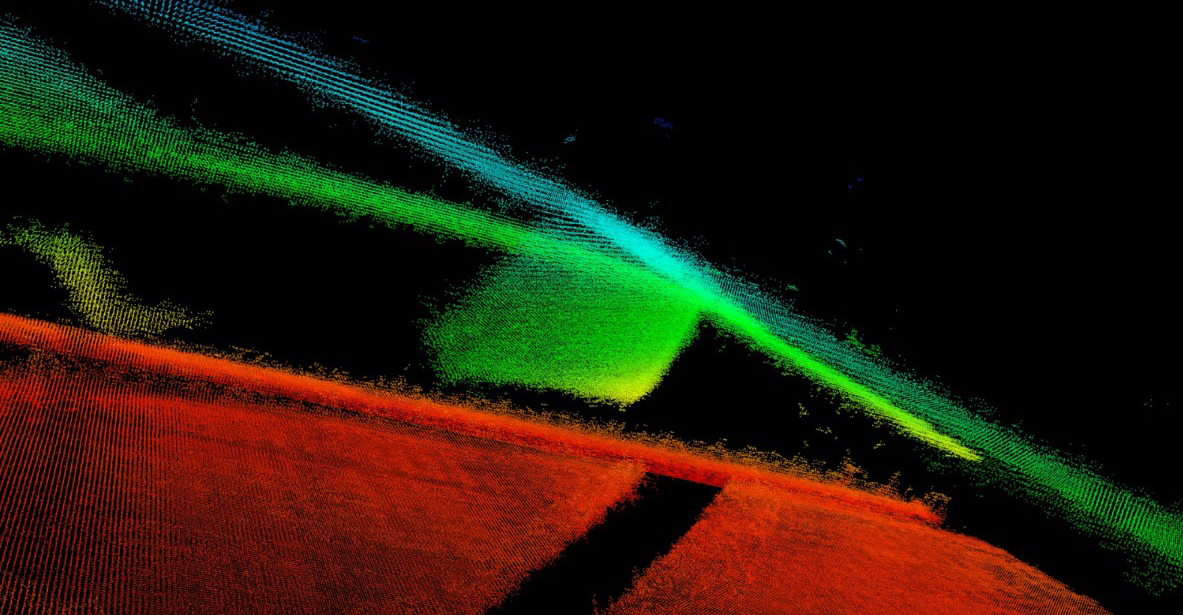
Figure 3: Overlay of Many Scans
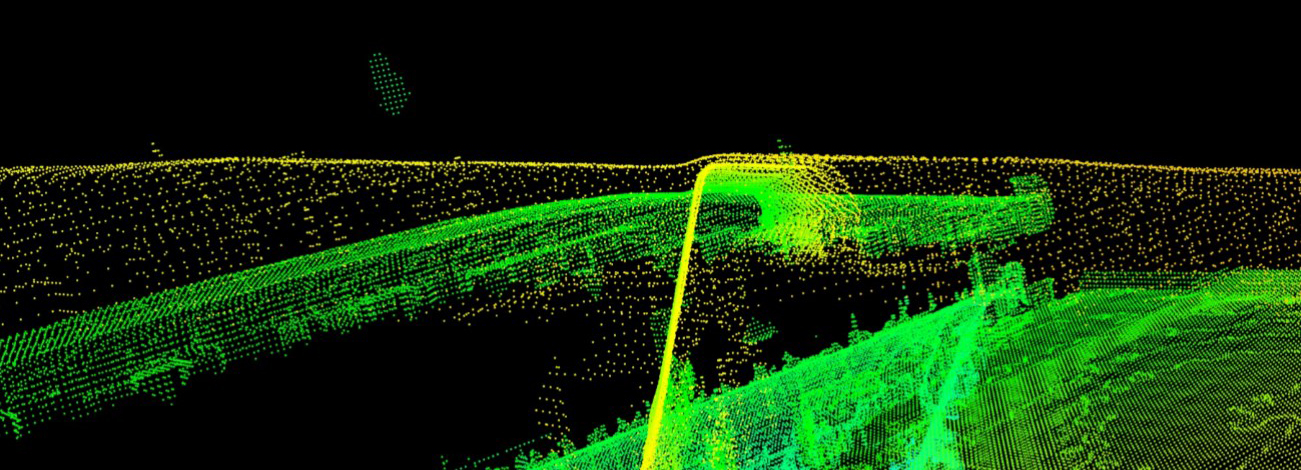
Figure 4: TSDF Detail View of Feature