Background
SwRI investigated the performance of algorithms applied to the output of a 77 GHz, high-resolution radar. Specifically, how well this class of radars can sense objects occluded by snow cover and to better understand terrain through varying depths of accumulated snow.
Approach
SwRI researchers modified their in-house Engineering Technology Demonstrator (ETD) platform to house a lidar and a new high-definition automotive radar. The researchers then set up and calibrated the lidar/radar onto a vehicle at a remote winter test facility in northern Michigan for the 2022/2023 winter season. Objects with known dimensions were placed in a test environment and natural snowfall was allowed to accumulate over several weeks. During this time, data was routinely collected. Another data collection was performed during the 2023/2024 winter season, which involved manually accumulating snow into specific depths to validate the radars capability of sensing objects beneath snow cover.
Accomplishments
SwRI concluded that while this class of radar would not generalize well for sensing obstacles beneath heavy snow cover, it does allow for very rapid, high-quality maps that can aid automated vehicle detection in environments where lidar and cameras may fail. SwRI researchers also created a novel addition to the Engineering Technology Demonstrator (ETD) harness that allowed for high-accuracy extrinsic sensor calibration between lidar and radar. The calibration procedure (for calibrating the radar with a lidar) was defined and tested while at SwRI headquarters and then was successfully performed at a remote test site. Data analysis algorithms were successfully developed and implemented to compute and overlay radar readings into globally accurate positions for accurate comparison across multiple data collections. Additional algorithms were developed that can readily convert the radar returns into usable datasets for segmentation and classification deep learning networks.
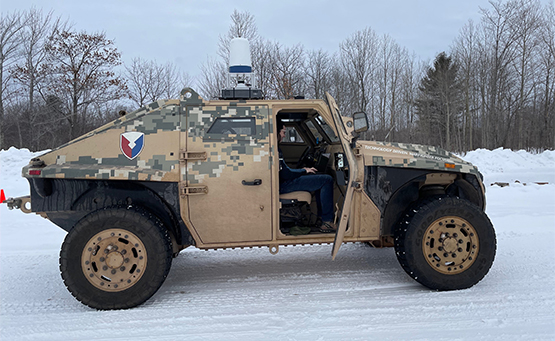
Figure 1: Modified ETD mounted to test vehicle at test site.
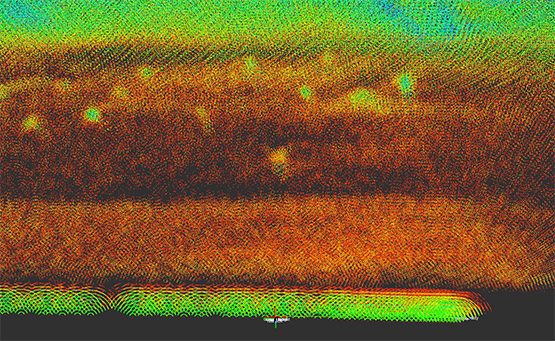
Figure 2: Accumulated and aligned radar point cloud returns.