Background
As part of an interdivisional effort, SwRI has developed a 3D markerless motion capture technology for human biomechanical assessment that provides measurement accuracies comparable to the industry standard marker-based motion capture systems. While this technology has proven accuracy and adaptability across many human movements and scenarios, it had previously only been deployed on human subjects. Within the zoological and animal health domain, being able to non-invasively observe and detect changes in non-human subjects’ health, activity levels and behavior would provide an invaluable early intervention tool. Conducting physical examinations of animal subjects, while a key component of animal care, is a costly procedure that interrupts the regular lives of the subjects and may not consistently provide accurate insights into the health of the subject. To assess the applicability of the SwRI markerless motion capture technology on non-human subjects, we partnered with Texas Biomedical Research Institute (TBRI) to observe an enclosure of baboons.
Approach
Our traditional data capture workflow was shifted from a single event-based trigger system to a continuous capture paradigm, requiring modifications to the existing camera launching software. Additionally, we developed a clear acrylic mounting panel that allowed the baboons to be recorded without the chain-link enclosure completely obscuring the foreground of the image.
Understanding that we would not be able to generate marker-based ground truth data, due to various problems with putting markers on baboons, including subject compliance, fur movement and environmental constraints, we further developed a labeling tool that allowed us to easily adjust and save joint locations of baboons in collected data. This labeled data would then get fed back into the neural network, allowing for increased auto-labeling accuracy. This iterative approach allowed for increased labeling speed as more data was collected.
We updated our neural network architecture to improve performance and modified the existing human labels to add in baboon keypoints (e.g., tail points). We performed an aggressive data augmentation strategy to overcome the relative scarcity of labeled data, implementing mirroring augmentation, brightness/contrast/hue-shift augmentation, and affine transform augmentation (varying scale, rotation, and translation). We employed the AdamW solver and trained our networks over 200 epochs (iterations over the entire training set). Figure 1 depicts a baboon pose as detected by our network.
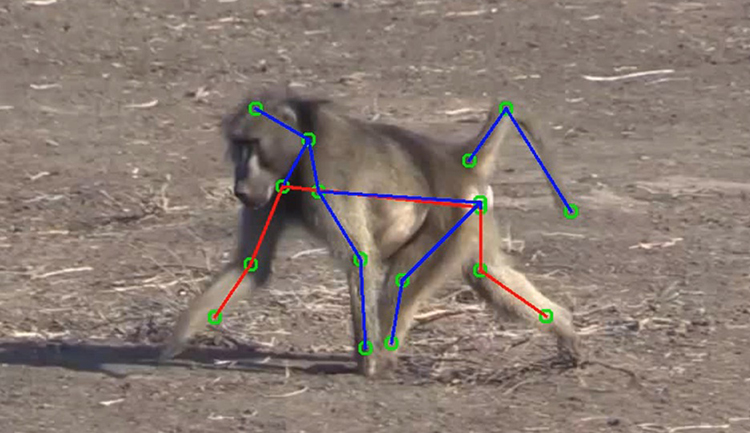
Figure 1: Baboon in the wild with detected keypoints from SwRI markerless motion capture technology.
Accomplishments
Our neural network results are presented in terms of a standard pose prediction metric, PCKh-0.5. Across all keypoints, our network had a PCKh-0.5 score of 89.2%. We speculate that this performance will improve with additional labeled data, but we were still able to attain good performance using thorough data augmentation procedures. Table 1 shows the performance of the network by keypoint type. Easily identifiable keypoints, such as the top of the head and base of the tail tend to score better, while more obscured keypoints or poorly defined landmarks (neck, hips) have lower scores.
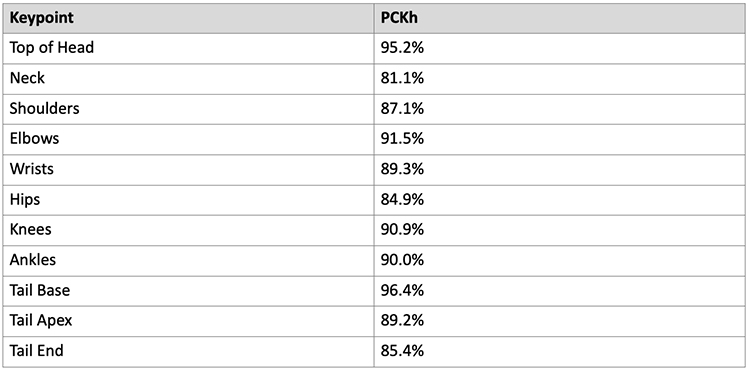
Figure 2: Neural network performance by Keypoint Type.